by Pati Ruiz and Judi Fusco
This glossary was written for educators to reference when learning about and using artificial intelligence (AI). We will start with a definition of artificial intelligence and then provide definitions of AI-related terms in alphabetical order. This glossary was last updated on March 31, 2024
Artificial Intelligence (AI): AI is a branch of computer science. AI systems use hardware, algorithms, and data to create “intelligence” to do things like make decisions, discover patterns, and perform some sort of action. AI is a general term and there are more specific terms used in the field of AI. AI systems can be built in different ways, two of the primary ways are: (1) through the use of rules provided by a human (rule-based systems); or (2) with machine learning algorithms. Many newer AI systems use machine learning (see definition of machine learning below).
________________________________________________
Algorithm: Algorithms are the “brains” of an AI system and what determines decisions in other words, algorithms are the rules for what actions the AI system takes. Machine learning algorithms can discover their own rules (see Machine learning for more) or be rule-based where human programmers give the rules.
Artificial General Intelligence (AGI): Artificial general intelligence has not yet been realized and would be when an AI system can learn, understand, and solve any problem that a human can.
Artificial Narrow Intelligence (ANI): AI can solve narrow problems and this is called artificial narrow intelligence. For example, a smartphone can use facial recognition to identify photos of an individual in the Photos app, but that same system cannot identify sounds.
Generative AI (GenAI): A type of machine learning that generates content, currently such as text, images, music, videos, and can create 3D models from 2D input. See ChatGPT definition, ChatGPT is a specific example of GenAI.
Chat-based generative pre-trained transformer (ChatGPT) models: A system built with a neural network transformer type of AI model that works well in natural language processing tasks (see definitions for neural networks and Natural Language Processing below). In this case, the model: (1) can generate responses to questions (Generative); (2) was trained in advance on a large amount of the written material available on the web (Pre-trained); (3) and can process sentences differently than other types of models (Transformer).
Transformer models: Used in GenAI (the T stands for Transformer), transformer models are a type of language model. They are neural networks and also classified as deep learning models. They give AI systems the ability to determine and focus on important parts of the input and output using something called a self-attention mechanism to help.
Self-attention mechanism: These mechanisms, also referred to as attention help systems determine the important aspects of input in different ways. There are several types and were inspired by how humans can direct their attention to important features in the world, understand ambiguity, and encode information.
Large language models (LLMs) Large language models form the foundation for generative AI (GenAI) systems. GenAI systems include some chatbots and tools including OpenAI’s GPTs, Meta’s LLaMA, xAI’s Grok, and Google’s PaLM and Gemini. LLMs are artificial neural networks. At a very basic level, the LLM detected statistical relationships between how likely a word is to appear following the previous word in their training. As they answer questions or write text, LLM’s use the model of the likelihood of a word occurring to predict the next word to generate. LLMs are a type of foundation model, which are pre-trained with deep learning techniques on massive data sets of text documents. Sometimes, companies include data sets of text without the creator’s consent.
Computer Vision: Computer Vision is a set of computational challenges concerned with teaching computers how to understand visual information, including objects, pictures, scenes, and movement (including video). Computer Vision (often thought of as an AI problem) uses techniques like machine learning to achieve this goal.
Critical AI: Critical AI is an approach to examining AI from a perspective that focuses on reflective assessment and critique as a way of understanding and challenging existing and historical structures within AI. Read more about critical AI.
Data: Data are units of information about people or objects that can be used by AI technologies.
Training Data: This is the data used to train the algorithm or machine learning model. It has been generated by humans in their work or other contexts in their past. While it sounds simple, training data is so important because the wrong data can perpetuate systemic biases. If you are training a system to help with hiring people, and you use data from existing companies, you will be training that system to hire the kind of people who are already there. Algorithms take on the biases that are already inside the data. People often think that machines are “fair and unbiased” but this can be a dangerous perspective. Machines are only as unbiased as the human who creates them and the data that trains them. (Note: we all have biases! Also, our data reflect the biases in the world.)1
Foundation Models: Foundation Models represent a large amount of data that can be used as a foundation for developing other models. For example, generative AI systems use large language foundation models. They can be a way to speed up the development of new systems, but there is controversy about using foundation models since depending on where their data comes from, there are different issues of trustworthiness and bias. Jitendra Malik, Professor of Computer Science at UC Berkeley once said the following about foundation models: “These models are really castles in the air, they have no foundation whatsoever.”
Human-centered Perspective: A human-centered perspective sees AI systems working with humans and helping to augment human skills. People should always play a leading role in education, and AI systems should not replace teachers.
Intelligence Augmentation (IA): Augmenting means making something greater; in some cases, perhaps it means making it possible to do the same task with less effort. Maybe it means letting a human (perhaps teacher) choose to not do all the redundant tasks in a classroom but automate some of them so they can do more things that only a human can do. It may mean other things. There’s a fine line between augmenting and replacing and technologies should be designed so that humans can choose what a system does and when it does it.
Intelligent Tutoring Systems (ITS): A computer system or digital learning environment that gives instant and custom feedback to students. An Intelligent Tutoring System may use rule-based AI (rules provided by a human) or use machine learning under the hood. By under the hood we mean the underlying algorithms and code that an ITS is built with. ITSs can support adaptive learning.
Adaptive Learning: Subject or course material is adjusted based on the performance of the learner. The difficulty of material, the pacing, sequence, type of help given, or other features can be adapted based on the learner’s prior responses.
Interpretable Machine Learning (IML): Interpretable machine learning, sometimes also called interpretable AI, describes the creation of models that are inherently interpretable in that they provide their own explanations for their decisions. This approach is preferable to that of explainable machine learning (see definition below) for many reasons including the fact that we should understand what is happening from the beginning in our systems, rather than try to “explain” black boxes after the fact.
Black Boxes: We call things we don’t understand, “black boxes” because what happens inside the box cannot be seen. Many machine learning algorithms are “black boxes” meaning that we don’t have an understanding of how a system is using features of the data when making their decisions (generally, we do know what features are used but not how they are used)There are currently two primary ways to pull back the curtain on the black boxes of AI algorithms: interpretable machine learning (see definition above) and explainable machine learning (see definition below).
Machine Learning (ML): Machine learning is a field of study with a range of approaches to developing algorithms that can be used in AI systems. AI is a more general term. In ML, an algorithm will identify rules and patterns in the data without a human specifying those rules and patterns. These algorithms build a model for decision making as they go through data. (You will sometimes hear the term machine learning model.) Because they discover their own rules in the data they are given, ML systems can perpetuate biases. Algorithms used in machine learning require massive amounts of data to be trained to make decisions.
It’s important to note that in machine learning, the algorithm is doing the work to improve and does not have the help of a human programmer. It is also important to note three more things. One, in most cases the algorithm is learning an association (when X occurs, it usually means Y) from training data that is from the past. Two, since the data is historical, it may contain biases and assumptions that we do not want to perpetuate. Three, there are many questions about involving humans in the loop with AI systems; when using ML to solve AI problems, a human may not be able to understand the rules the algorithm is creating and using to make decisions. This could be especially problematic if a human learner was harmed by a decision a machine made and there was no way to appeal the decision.
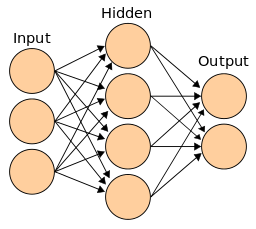
Illustration of the topology of a generic Artificial Neural Network. This file is licensed under the Creative Commons Attribution-Share Alike 3.0 Unported license.
Neural Networks (NN): Neural networks also called artificial neural networks (ANN) and are a subset of ML algorithms. They were inspired by the interconnections of neurons and synapses in a human brain. In a neural network, after data enter in the first layer, the data go through a hidden layer of nodes where calculations that adjust the strength of connections in the nodes are performed, and then go to an output layer.
Deep Learning: Deep learning models are a subset of neural networks. With multiple hidden layers, deep learning algorithms are potentially able to recognize more subtle and complex patterns. Like neural networks, deep learning algorithms involve interconnected nodes where weights are adjusted, but as mentioned earlier there are more layers and more calculations that can make adjustments to the output to determine each decision. The decisions by deep learning models are often very difficult to interpret as there are so many hidden layers doing different calculations that are not easily translatable into English rules (or another human-readable language).
Natural Language Processing (NLP): Natural Language Processing is a field of Linguistics and Computer Science that also overlaps with AI. NLP uses an understanding of the structure, grammar, and meaning in words to help computers “understand and comprehend” language. NLP requires a large corpus of text (usually half a million words).
NLP technologies help in many situations that include: scanning texts to turn them into editable text (optical character recognition), speech to text, voice-based computer help systems, grammatical correction (like auto-correct or grammarly), summarizing texts, and others.
Robots: Robots are embodied mechanical machines that are capable of doing a physical task for humans. “Bots” are typically software agents that perform tasks in a software application (e.g., in an intelligent tutoring system they may offer help). Bots are sometimes called conversational agents. Both robots and bots can contain AI, including machine learning, but do not have to have it. AI can help robots and bots perform tasks in more adaptive and complex ways.
User Experience Design/User Interface Design (UX/UI): User-experience/user-interface design refers to the overall experience users have with a product. These approaches are not limited to AI work. Product designers implement UX/UI approaches to design and understand the experiences their users have with their technologies.
Explainable Machine Learning (XML) or Explainable AI (XAI): Researchers have developed a set of processes and methods that allow humans to better understand the results and outputs of machine learning algorithms. This helps developers of AI-mediated tools understand how the systems they design work and can help them ensure that they work correctly and are meeting requirements and regulatory standards.
It is important to note that the term “explainable” in the context of explainable machine learning or explainable AI, refers to an understanding of how a model works and not to an explanation of how the model works. In theory, explainable ML/AI means that an ML/AI model will be “explained” after the algorithm makes its decision so that we can understand how the model works. This often entails using another algorithm to help explain what is happening as the “black box.” One issue with XML and XAI is that we cannot know for certain whether the explanation we are getting is correct, therefore we cannot technically trust either the explanation or the original model. Instead, researchers recommend the use of interpretable models.
Thank you to Michael Chang, Ph.D., a CIRCLS postdoctoral scholar, for reviewing this post and to Eric Nentrup for support with specific definitions. We appreciate your work in AI and your work to bring educators and researchers together on this topic.
________________________________________________
1Fusco, J. (2020). Book Review: You Look Like a Thing and I Love You. CIRCLEducators Blog. Retrieved from https://circleducators.org/review-you-look-like-a-thing/