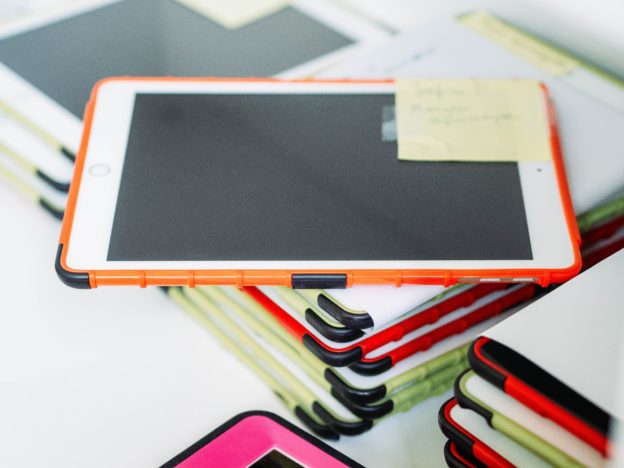
image by Arthur Lambillotte via Unsplash
by Courtney Teague, Rita Fennelly-Atkinson, and Jillian Doggett
Courtney Teague, EdD, Deputy Director of Internal Professional Learning and Coaching with Verizon Innovative Learning Schools program based in Atlanta, GA.
Rita Fennelly-Atkinson,EdD, Director Micro-credentials with the Pathways and Credentials team based in Austin, TX
Jillian Doggett M.Ed, Project Director of Community Networks with Verizon Innovative Learning Schools program based in Columbus, OH
What is microlearning?
Microlearning is a teaching and learning approach that delivers educational content in short, focused bursts of information. Microlearning tends to focus on one objective, and the learning doesn’t require more than 1-20 minutes of the learner’s time. Schools and teachers can use microlearning to supplement traditional instruction or as a standalone learning tool. microlearning has been around for a long time–remember those flashcards at kindergarten that helped us learn numbers, the alphabet, and colors? However, schools were largely unaware of how powerful this learning strategy can be for a teacher.
Microlearning has several potential benefits for both learners and teachers. For learners, microlearning can provide a more engaging and interactive learning experience. This type of instruction can also help to reduce distractions for students who become disengaged with unnecessary learning information. For teachers, microlearning can be used to differentiate instruction and address the needs of all learners. Additionally, microlearning can save instructional time by allowing teachers to deliver targeted information in a concise format. Teachers can tailor microlearning content to focus on specific skills or knowledge gaps (Teague, 2021).
Microlearning is flexible and can be accessed anytime, anywhere. Learners can complete microlearning activities on their own time, at their own pace. Microlearning is like a seasoning for learning; it seasons and heats up information to make the process of comprehending new knowledge easier. It has been part-and-parcel in many schools’ instructional strategies since time immemorial, but only recently have we begun paying attention to how powerful this strategy really can be when used correctly.
What does microlearning look like?
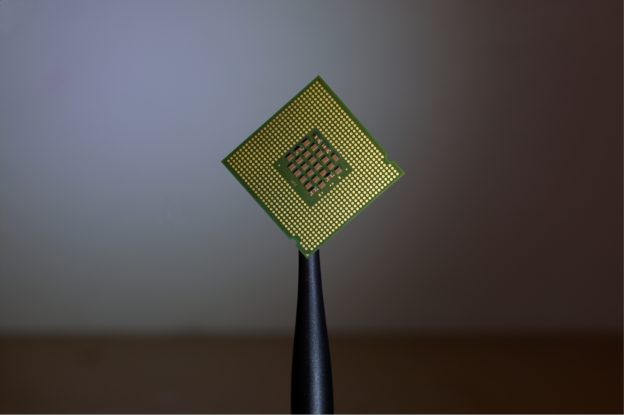
image by Brian Kostiuk via Unsplash
Microlearning can come in many forms. Below is a list of 10 microlearning examples:
- Short, Focused Videos
- Infographics
- Podcasts or Audio Recordings
- Social Media Posts and Feeds
- Interactive Multimedia
- Animations
- Flashcards
- Virtual Simulations
- Assessment Activities: Polls, Multiple-Choice Questions, Open Response Questions
- Games
How can teachers use microlearning effectively to maximize content retention, personalize learning experiences, and bolster student engagement?
Use microlearning to Active Student Prior Knowledge and Generate Excitement for New Learning
Assign microlearning, such as a self-paced learning game, to assess and activate prior knowledge around a topic. Or place a few bite-sized learning opportunities about an upcoming lesson in your Learning Management System (LMS) for learners to preview beforehand to generate interest and excitement for new learning.
Use microlearning to Personalize Learning Experiences
Creating microlearning in various formats covering multiple topics gives learners the agency to make meaningful choices about their learning paths. For example, to learn a new concept or build new skills, learners can choose to engage with an interactive image, listen to a short audio guide, participate in a learning game, or watch an explainer video or animation. Additionally, learners who need remediation or want to extend their learning can quickly access content to review a topic again or complete additional microlearning lessons.
Use microlearning to Encourage Communication and Collaboration
Create different microlearning bites, each covering a specific objective or portion of a learning goal. Assign each student to engage with one microlearning bite and then use the Jigsaw method to have learners learn about a new topic in a cooperative style. Similarly, you can assign microlearning that includes thought-provoking, probing questions and have learners discuss on a discussion forum or by recording and responding to each other’s short video or audio responses.
Use microlearning to Engage Families and Caretakers
Distribute microlearning to learners’ families and caretakers to help them quickly learn content learners are learning in class to support them in taking an active role in their child’s learning at home.
Use microlearning to Reduce Time Spent Grading
Create microgames and assessments using tools that automatically grade and provide learner analytics to reduce the time spent grading. For example, create an interactive video with embedded questions, a short quiz on your LMS, or a learning game that automatically grades learners’ responses and provides you with learner analytics you can use right away to inform just-in-time teaching.
Use microlearning to Build Classroom Community
Use microlearning to Promote Learning Outside of SchoolOver time, create and curate a repository of microlearning assets, such as explainer videos, audio recordings, infographics, learning games, trivia quizzes, flashcards, etc., on your Learning Management System (LMS). Then, learners can easily access and continue their learning outside of school, cultivating a life-long learning mindset.
How to assess microlearning?
The flexibility of microlearning allows for an abundance of possibilities in how it is assessed. For example, if your goal is simply to educate people about a new process using a video, then you don’t have to assess, you can simply measure the reach by the number of views and effectiveness by the level of adherence to the new process by a specific date. If your goal is to educate people about the available services, then your performance indicator might be the use of those services. In other words, you have a license to be creative and to assess learning effectiveness in many different ways.
More formally, the evaluation of learning can be categorized into two types: assessments and indicators (Fennelly-Atkinson & Dyer, 2021). Assessments include most formal and informal methods of evaluating learning, which include surveys, check-ins (i.e. verbal, data, progress, etc), completion rates, knowledge checks, skill demonstration observations, self-evaluations, and performance evaluations. Meanwhile, indicators include indirect measures such as performance, productivity, and success benchmarks. Which type you use is largely dependent on the learning context and need. The key questions to consider are the following:
- What measurable change is the microlearning impacting?
- Do you need individual, organizational, or both types of data?
- What is the ease of collecting and analyzing the data?
- Can existing evaluations or indicators be used to measure the impact of learning?
What are the drawbacks of microlearning & how to mitigate them?
Microlearning does have some potential drawbacks. For one thing, it can be easy for learners to become overwhelmed by the sheer volume of micro-lessons that they are expected to complete. Additionally, microlearning can sometimes result in a fragmented understanding of a topic, as learners are only exposed to small pieces of information at a time. Microlearning often does not provide an opportunity for learners to practice and apply what they have learned. However, these potential drawbacks can be avoided or mitigated when microlearning is designed into learning activities. Another potential drawback of microlearning is that it can be difficult to maintain a consistent level of quality control. With so much content being produced by so many different people, it can be hard to ensure that all of the material is accurate and up to date. This problem can be mitigated by careful selection of materials and regular quality checks. Because of this, microlearning can create a significant amount of work for teachers. In order to properly incorporate microlearning into their classrooms, teachers need to have a good understanding of the material and be able to effectively facilitate discussion and debate. While it may require some additional effort on the part of teachers to do microlearning, it feels worth it as it has the potential to significantly improve student engagement and learning outcomes.
Which tools can you use to create microlearning?
While microlearning does not necessarily require the use of digital tools, the reach and potential of these types of learning experiences is magnified by technology. Because microlearning is so short and usually discrete, there are many types of tools and methods of delivery that can be used. Formal authoring tools such as LMSs and Articulate can be used, but are not required. Any type of tool that can create a static or dynamic piece of content can be used. Further, any type of delivery system can be used to disseminate the learning. Making microlearning relevant and specific to the learning context, environment, and audience are key to selecting a content creation tool and delivery systems (Fennelly-Atkinson & Dyer, 2021).
Summary
To wrap it up, microlearning is breaking down and chunking learning into bite-sized pieces. Microlearning might be small but can have a big impact on powerful teaching and learning. It can take many different forms, which means that there are just as many content-creation tools and delivery platforms. Likewise, there are a variety of ways to assess microlearning depending on the goal and purpose for its use. There is no one correct way of creating microlearning. Microlearning can be as simple as listening to the pronunciation of words on an audible dictionary online application. Teachers can use this flexible method of microlearning to support research-based instructional practices and personalize learning experiences.
So how might you use this approach to meet the modern learner’s needs? Tweet @EducatorCIRCLS and be part of the conversation.
References
Fennelly-Atkinson, R., & Dyer, R. (2021). Assessing the Learning in microlearning. In Microlearning in the Digital Age (pp. 95-107). Routledge.
Teague, C. (2021, January 11). It’s All About microlearning. https://community.simplek12.com/webinar/5673